
Machine learning is a self-learning tactic to train the model, but its training phase is the most load-intensive one where the process takes the longest span, and an average computer can’t execute so much load. You may have to wait for hours or even weeks to complete the training phase, which diminishes productivity by extending and wasting time. There are several ways to reduce such efficiencies. One is choosing the best GPU for machine learning and deep learning to save time and resources. A graphics card powers up the system to quickly perform all the load-taking tasks by cooperating with the processor.
A good-quality graphics card reduces latencies, improves overall efficiency, and optimizes target tasks to speed up the training process. With an excellent GPU, you won’t have to wait for hours to see if the models have trained, so we can pursue working on it. Instead, you will complete the operation and even multiple functions simultaneously. It’s easy to understand the importance of a graphics card for machine learning, but quite tricky to find one when there are so many in the market. But this post can help you in the process. We have tested and reviewed some impressive graphics cards, so check the whole list to choose one.
Quick Shopping Tips
Brand: There are two primary brands when it comes to graphics cards, Nvidia and AMD. But, some third-party brands are also there who just take some original cards from the duo and re-design them. Nvidia is good for gaming and overclocking, while AMD’s cards are perfect for multi-tasking and productivity tasks. Other third-party brands like ASUS, XFX, MSI, and more are also great. I suggest you go for a good-quality card rather than a brand name.
VRAM size: Like the volatile memory, the graphics unit has a particular amount of memory called VRAM or video memory. The higher the total memory, the higher the system’s overall performance. Your system must have at least a 4GB graphics card for limitations brackets, but there is no such upper limit; you can go as high as possible. Remember, you must explore your usage and purchase a graphics card according to the requirement.
Form Factor: Graphics cards are available in many sizes, each with separate dimensions and accommodations. Remember, if you buy a card with the wrong size according to the PC case and motherboard, it will not fit properly and can’t be used as expected. So, make sure you take the measurements of every corner where you will hold the graphics card and buy one according to those dimensions.
Supporting software: In machine learning, trainers don’t directly program but need to run many to properly train and execute the instructions. You must ensure that the card supports machine learning libraries and some common frameworks such as PyTorch or TensorFlow. NVIDIA GPUs support all those more often since the CUDA toolkit has some GPU-accelerated libraries such as C, C++ compilers, and more.
Clock speed: The clock speed or frequency is the speed of the graphics card. It is directly proportional to the performance since it reduces the execution time and boosts the system’s efficiency. In addition, every card has a particular base clock speed that can increase up to a certain level of boost speed, creating overclocking headroom. You must prefer a card with higher overclocking headroom and overall clock speeds.
Thermal design: The thermal design of the graphics card is also important in determining the overclocking and performance. Most of the time, we see simple fans enabling airflow through the card’s body and dissipating the heat faster. But the number of fans, design and air-carrying capacity may vary depending upon the card and your PC design. So when purchasing a graphics card, you have to check the cooling design and go for a card with excellent cooling performance.
The Best GPU for Machine and Deep Learning You Can Buy Today
Award | Design | Best | Retailer |
---|---|---|---|
Best Premium GPU for Machine Learning | ![]() | View | |
Best High-End GPU for Machine Learning | ![]() | View | |
Best Budget-Friendly GPU for Machine Learning | ![]() | View | |
Best Flagship GPU for Machine Learning | ![]() | View | |
Best Entry-Level GPU for Machine Learning | ![]() | View | |
Best Performance GPU for Machine Learning | ![]() | View | |
Best Affordable GPU for Machine Learning | ![]() | View | |
Best Selling GPU for Machine Learning | ![]() | View |
NVIDIA GeForce RTX 3080 Founders Edition
Best Premium GPU for Machine Learning
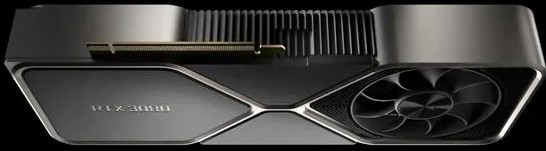
Brand: Nvidia | Series/Family: GeForce RTX 3000 series | GPU: Nvidia Ampere GA102 | GPU architecture: Nvidia Ampere architecture | Memory: 10GB GDDR6X | Memory bus: 320-bit | Memory speed: 19Gb/s | Cache: Unknown | Base Clock: 1365MHz | Game clock: Unknown | Boost clock: 1665MHz | Power Consumption: 350W | Cooling fans: Dual 85mm Axial fans
REASONS TO BUY
✓Tough build
✓Decent design
✓Excellent cooling
✓Two HDMI 2.1 ports
REASONS TO AVOID
✗Highest TDP for a single GPU
NVIDIA keeps improving its previous technologies and discovering new ones to match up with the tech requirements. We can see the same mastery in its RTX 3000 series, which carries numerous advancements to achieve 50% more performance than the previous RTX 2000 series. The NVIDIA GeForce RTX 3080 is the first third-party addition to the series and the second most-fastest card after the RTX 3090 graphics card. It’s a perfect mixture of high-end performance, the fastest speeds, and excellent thermal management to reach its peak efficiency level.
Like the other advanced Nvidia graphics cards, this one is powered by the Ampere architecture along with second-generation Ray Tracing and third-generation Tensor cores. Moreover, it has 8704 CUDA cores and 10GB of GDDR6x memory with 19Gb/s memory speed based on a 320-bit memory bus. You can utilize three DisplayPort and two HDMI 2.1 ports to support multi-monitor features to power four monitors simultaneously. The RTX 3080 is the best premium GPU for machine learning since it’s a perfect match to reduce the latencies while training the model.
It seems that ASUS’s designers have spent hours designing and manufacturing the card and embedding the military-grade components on the PCB sheet. The high-quality chokes, premium MOSFETs, and durable capacitors are combined to provide a longer life to the product. If we look at the card, it has a unique look, a black mixed with silver accent, and the model name written on the front panel. Moreover, the brand has installed an excellent cooling design having three axial-tech fans, a massive heatsink, and six heat pipes for thermal management.
NVIDIA GeForce RTX 2080 Ti
Best High-End GPU for Machine Learning

Brand: Sapphire | Series/Family: Pulse RX 5000 series | GPU: TU102 | GPU architecture: Nvidia Turing architecture | Memory: 11GB GDDR6 | Memory bus: 352-bit | Memory speed: 14000MHz | Cache: Unknown | Base Clock: 1350MHz | Game clock: Unknown | Boost clock: 1545MHz | Power Consumption: 260W | Cooling fans: Dual 13-blade fans
REASONS TO BUY
✓Fastest for machine learning
✓Silent fans movement
✓Excellent cooling
✓Energy-efficient
REASONS TO AVOID
✗Pricey
✗Only one HDMI port
NVIDIA is the most-heard name in the graphics cards market with the most customers on its side. We have the NVIDIA GeForce RTX 2080 Ti, which is only 1% behind the next-generation RTX 3080 and is way faster than the RTX 2080 variant in the series. The all-new Turing architecture powers it with the advanced RTX platform and a wide range of modern technologies. You can enjoy the spices of faster performance, real-time ray tracing, AI-based methodology, and more with this best high-end GPU for machine learning and get the most out of any training system and model.
If we uncover the specification chart, the card uses a TU102 graphics unit with 11GB GDDR6 memory is enough to deliver 616 GB/s bandwidth and 14 Gb/s memory speed with a 352-bit memory bus. You can take its 1350MHz base clock up to a 1545MHz boost clock to tackle a wide range of loads while training the models. Other important factors include 4352 CUDA core processors, 76T RTX OPS, 96 ROP units, 72 Ray Tracing cores, and 576 Tensor cores. In short, there is pretty much everything you may need during your ML processes and high-end gaming sessions.
Although the chip is made for high-end gaming performance, it can help you train the models in the same way since both tasks use the same technologies. It will save you time and energy, plus it will take all the multitasking responsibilities on itself. The card adorns a premium look with a brushed metal backplate for protection but a plastic front panel. Other than that, its efficient cooling system empowers you to perform all types of tasks. It uses a Tri-FROZR cooler design with three fans featuring a unique fan-blade design to effectively deliver theirs to the massive heatsink.
Sapphire Radeon Pulse RX 580
Best Budget-Friendly GPU for Machine Learning
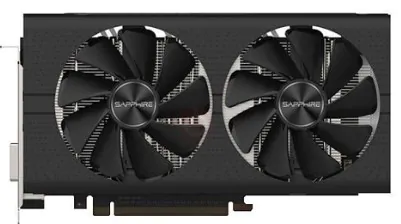
Brand: Sapphire | Series/Family: Pulse RX 500 series | GPU: Polaris GPU | GPU architecture: 4th-generation Graphics Core Next architecture | Memory: 8GB GDDR5 | Memory bus: 256-bit | Memory speed: 8000 MHz | Cache: Unknown | Base Clock: 1217MHz | Game clock: Unknown | Boost clock: 1340MHz | Power Consumption: 185W | Cooling fans: Dual 92mm fans
REASONS TO BUY
✓Affordable
✓Competitive performance
✓Power-efficient
✓Adequate thermal management
REASONS TO AVOID
✗No DVI-D port
Sapphire has been in the market for years with record-breaking inventions that led it to get a long name, especially when it comes to manufacturing AMD graphics cards. We have added many famous RX 580s in many of our reviews that were quite famous for excellent performance via many outstanding features. However, the story of the Sapphire Radeon RX 580 is a bit different since it’s renowned for having the most attractive price for tight-pocket users. The title of the best budget-friendly GPU for machine learning sits totally valid when it delivers performance like the expensive Nitro+ cards.
The card is powered by the Polaris graphics architecture, the 4th generation GCN architecture with some future-proof technologies. If you look at the specifications chart, it has 8GB GDDR5 memory that can effectively reach up to 8000MHz memory speed, 2304 stream processors, and a 1366MHz of engine clock speed. In addition, the engine speed can go up to 1411MHz when you shift to silent mode powered by stable power, adequate cooling, and other features. Overall, the card is an excellent choice for those who want enough performance but at less price.
Want to know its graphics performance? Well, it suits perfectly to deliver 1080p to 1440p graphics resolution depending upon the game and other graphics-related settings. As far as connectivity matters, there are three DisplayPorts and one HDMI port to utilize the card’s capabilities. The card is 9.5 inches in length and has a rugged aluminum-made backplate to help with protection and cooling. It features the Sapphire Dual-X cooling design, famous for its excellent airflow and thermal management, containing two 92mm fans with dust-repelling dual ball bearings.
NVIDIA GeForce RTX 2070
Best Flagship GPU for Machine Learning

Brand: Nvidia | Series/Family: GeForce RTX 2000 series | GPU: TU106 | GPU architecture: Nvidia Turing architecture | Memory: 8GB GDDR6 | Memory bus: 256-bit | Memory speed: 14Gb/s | Cache: Unknown | Base Clock: 1410MHz | Game clock: Unknown | Boost clock: 1620MHz | Power Consumption: 175W | Cooling fans: Dual fan setup
REASONS TO BUY
✓Outstanding synthetic performance
✓Plays 4k games
✓Competitively power-efficient
✓DLSS and Ray Tracing support
REASONS TO AVOID
✗No SLI support
The NVIDIA GeForce RTX 2070 delivers the ultimate performance being a mainstream Turning GPU and replaces the previous RTX 2060 series. You can expect an impressive level of speed and lower latencies when training your model during the machine learning sessions. Moreover, if you can’t afford the pricey RTX 2080 Ti, this best flagship GPU for machine learning must be your first choice with similar performance benchmarks. The card is powered by the NVIDIA Turning architecture combined with the RTX platform and AI-based technologies to adequate power to train AI models.
It’s 100% convincing when the real-time Ray Tracing performance hits the 6 Giga Rays per second mark. You may say it is a downgraded version of the RTX 2080 with a TU106 processing chip and several other impressive aspects. It has the same 8GB GDDR6 memory with 14 Gb/s memory speed and 448 GB/s memory bandwidth with a 256-bit memory bus. You can take its engine’s 1410MHz base clock up to a 1620MHz boost clock. The card has 2304 CUDA cores which are slightly lower than 4352 in RTX 2080 but still acceptable since this card is from the previous generation.
You can support up to four monitors with the card’s multi-monitor support and connect those with DisplayPort, HDMI, and DVI-D–an USB Type-C port is also given. The design presents a metallic look both in the form and in the back, decorated with a silver accent and lined-backplate at the back. Interestingly, the brand has redesigned the structure and installed a 6-phases power supply with two 13-blade fans grouped with a vapor chamber for quiet and ultra-cooling performance. Overall, the card has the same price as the GTX 1080 but offers way more performance with ray tracing.
MSI Gaming GeForce GT 710 2GB
Best Entry-Level GPU for Machine Learning
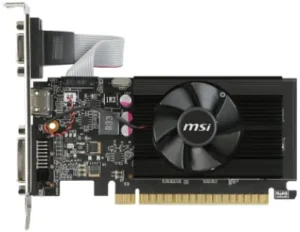
Brand: MSI | Series/Family: Unknown | GPU: GK208 | GPU architecture: Kepler GPU architecture | Memory: 2GB GDDR3 | Memory bus: 64-bit | Memory speed: 1600MHz | Cache: Unknown | Core Clock: 954MHz | Power Consumption: 19W | Cooling fans: Single fan setup
REASONS TO BUY
✓Affordable
✓Perfect for beginners
✓Easy to install
REASONS TO AVOID
✗Just enough performance
✗DDD3 memory
If you’re just stepping into the world of machine learning and deep learning, the MSI Gaming GeForce GT 710 is for you. It was launched back in 2014, and this date makes it slightly outdated, but it’s still the best entry-level GPU for machine learning. So, if you want some extra output from the existing PC, you should definitely try this graphics card. You can add up to three displays and use NVIDIA’s driver support, enhanced performance, some good-looking specifications, and a few impressive technologies. Moreover, it works soundlessly and can fit into all types of hardware without any issues.
Honestly, I wasn’t expecting much from an entry-level graphics card but it surprises us with a wide range of excellent features. The GT 710 is based on the old GK208 chip powered by Kepler graphics architecture. It is manufactured on a 28nm TSMC processor and 768 CUDA cores, and a 2GB DDR3 type memory with 1600MHz memory speed based on a 64-bit memory interface. Apart from that, its engine can operate with a 954MHz core clock which is not like the current-era cards but complements the product’s price. In short, it can’t compete with the other cards on the list but is perfect as a starting pack.
There are nine sensors to keep the monitor memory and VRM in check so you can take it up to its maximum performance level with this best GPU for machine learning and deep learning. You can connect three monitors simultaneously to get power from the same card with the help of one display port, one HDMI connection, and a DVI-D slot. If we compare its 5.75 x 0.75 x 2.72 inches dimensions with the others in the market, the card falls in the compact category to build a smaller system. It occupies two expansion slots with such a size plus easy to install with a smaller form factor saving you time.
ASUS ROG Strix AMD Radeon RX 5700XT OC
Best Performance GPU for Machine Learning
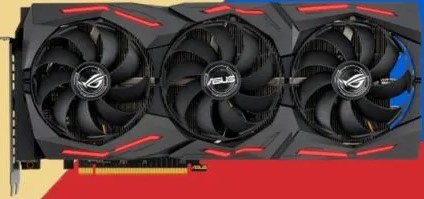
Brand: ASUS | Series/Family: ROG Strix | GPU: Navi 14 GPU unit | GPU architecture: Graphics Core Next 1.3 architecture | Memory: 8GB GDDR5 | Memory bus: 256-bit | Memory speed: 7000MHz | Cache: Unknown | Base Clock: 1178MHz | Game clock: 1244MHz | Boost clock: 1254MHz | Power Consumption: 187W | Cooling fans: Dual fan setup
REASONS TO BUY
✓Good value for money
✓Packed with good-quality features
✓Faster than the ancestors
✓Operates quietly
REASONS TO AVOID
✗No NVlink support
At the end of the reviews section, we have the ASUS Rog Strix Radeon RX 5700XT, which is the best performance GPU for machine learning and deep learning due to a number of reasons. It is the top-tier version in the series made for delivering higher performance and higher-end specifications. It has higher processing power, excellent boosting technology, faster memory, and more. If we compare it with the previous Strix RX 470 OC, the brand has intelligently improved its cooling solution, card layout, and overclocking since some users have faced some issues with the RX 470 OC.
If we look at the inside body of the card, it uses a Navi 14 graphics unit packed with 8GB GDDR5 memory that can run with 7000MHz memory speed and 224 Gb/s bandwidth with the help of a 256-bit memory bus. It has 2048 stream processors, a 1737MHz base clock speed that can increase to a 1845MHz boost clock, and more at one spot. Interestingly, it supports connecting up to six monitors simultaneously and has one HDMI 2.0 port, one DisplayPort 1.4, and a pair of native DVI-D connections. Overall, you get all the essential specifications that a current-era card must have.
As far as the performance matters, it can deliver up to 1080p to 1440p high-quality graphics in major AAA titles games, plus it can help you boost up the machine learning process reducing the latencies—it’s not a good option for 4k enthusiasts. Remember, the card is built on a multi-layer PCB sheet along with high-quality MOSFETs, chokes, and other power elements to deliver optimal input power for efficient performance. Look-wise, it has RGB lighting decorating a black accent painted on a uniquely designed body having fans with two different logos in the center of each.
MSI Gaming GeForce GTX 1660 Super Ventus XS
Best Affordable GPU for Machine Learning

Brand: MSI | Series/Family: Gaming Ventus XS | GPU: TU116 | GPU architecture: Nvidia Turing architecture | Memory: 6GB GDDR6 | Memory bus: 192-bit | Memory speed: 14Gb/s | Cache: Unknown | Base Clock: 1815MHz | Game clock: Unknown | Boost clock: 1815MHz | Power Consumption: 125W | Cooling fans: Dual fan setup
REASONS TO BUY
✓Affordable
✓Overclocking and performance
✓Effective thermal management
✓Attractive design with slight RGB touch
✓Low-noise fans
REASONS TO AVOID
✗RTX and DLSS feature is not included
We have done deep research before writing this article and discovered some helpful pieces of information related to the tech market. Most importantly, a majority of the customers want a budget-friendly and mid-range type of product with good performance. If you’re one from such a group, the MSI Gaming GeForce GTX 1660 Super is the best affordable GPU for machine learning for you. It delivers 3-4% more performance than NVIDIA’s GTX 1660 Super, 8-9% more than the AMD RX Vega 56, and is much more impressive than the previous GeForce GTX 1050 Ti GAMING X 4G.
It has a TU116 Graphics Processing Unit powered by the advanced Turing graphics architecture. The specifications sheet says that the card has 6GB GDDR6 type memory that can run with 14Gb/s memory speed based on a 192-bit memory interface. Also, it has 1408 CPU core processors, and its engine clock speed can go up to 1830MHz boost clock speed. If you use the multi-monitor support correctly, you can connect four displays simultaneously with the help of three DisplayPorts and one HDMI connection.
It not only plays well in the gaming session but can perform impressively to help your system train the models during deep learning and machine learning tasks. Undoubtedly, it will win your heart with exceptional performance and a reachable price tag. At the end of the review, I would like to add some light on its design and cooling structure. The card features a black look painted with a gunmetal gray color supported by a classy brushed metal backplate mixed with RGB lighting. The Twin Frozr 7 thermal design contains two fans, intelligent aerodynamics, and a massive heatsink.
ZOTAC GeForce GTX 1070 Mini
Best Selling GPU for Machine Learning

Brand: Zotac | Series/Family: GeForce GTX 1000 series | GPU: GP104 | GPU architecture: Nvidia Pascal architecture | Memory: 8GB GDDR5 | Memory bus: 256-bit | Memory speed: 8GHZ | Cache: Unknown | Base Clock: 1518MHz | Game clock: Unknown | Boost clock: 1708MHz | Power Consumption: 150W | Cooling fans: Dual fan setup
REASONS TO BUY
✓Excellent performance
✓Overclocking
✓Adequate thermal management
✓VR-ready graphics card
✓Noiseless fans’ movements
REASONS TO AVOID
✗Relatively pricey
Starting reviewing the list with the Zotac GeForce GTX 1070 Mini, an incredible addition to the tech market with enthusiast features and specifications. The GTX 10 series is built around the advanced NVIDIA Pascal architecture, so without missing a beat, it also uses the same empowering engine. I’m sure you know about this graphics architecture. If not, then listen; it’s an efficient and power-efficient design, and you don’t even have to use an insane cooling setup since it can self-cool itself with excellent thermal management. All these are enough to call it the best GPU for machine learning, deep learning, and more.
The card uses the GP104 graphics unit, famous for its performance mixed with superb features, including immersive VR support. It’s more like the reference higher-end NVIDIA Founder Edition but with some alterations. You get 8GB GDDR5 memory with an 8GHz memory clock carved on a 256-bit memory interface. It has 1920 CUDA cores and a 1506MHz that can bump up to a 1683MHz boost clock. To connect it, you can use three DisplayPorts 1.4, one HDMI 2.0b slot, and DL-DVI graphics output.
Interestingly, you can power four displays at a time with this card for multi-monitor support and VR setup. If we unfold the performance statistics, it can deliver 1440 graphics quality with 90+ fps and 4k with 60+ fps resolution–the reason why it is the best selling GPU for machine learning. It has an attractive majorly black color with some grey lining on the fan holders’ sides carved on an attractively bent structure. For cooling purposes, there are three 90mm EKO fans that rotate silently to not disturb you during your training sessions, an Ice Storm cooler, six copper heat pipes, and a wrap-around metal backplate.
Conclusion
Machine learning puts an intense load on a system when training the model. The process can take hours and even days to feed all the data into the model and execute that to get the output. However, we can increase overall productivity by putting the best GPU for machine learning into the system and reducing the time. A graphics unit takes the graphics load from the processor and separately performs its functionalities to compute the data in no time. Indeed. If you’re really into machine learning, having an excellent card is your responsibility.
It’s easy to know that you need a graphics card but very complicated to find one of the best Graphics cards for machine learning. You need to put a reasonable amount of time into uncovering a compatible graphics card for your system. However, we have tried our best to introduce you to at least one best-suiting product to you, fulfilling all your needs. The buying guide section at the beginning of the article helps you understand some must-have factors in the compatible card. On top of that, the list contains all types of products for every user’s needs. So find your pain points and start seeing the best product according to the usage.
Frequently Asked Questions
Should I buy a GPU for deep learning?
The short answer is yes. You must invest some bucks into a good-quality graphics card to support the model’s learning process. It helps in reducing latencies, enhancing efficiency, and bringing the performance up to an optimal level.
Is a 2GB GPU enough for deep learning?
The VRMA size depends upon your usage, but still, 2GB is too less. You must invest in at least 4GB of graphics memory if you want to train your model in a reasonable time. Additionally, if you go beyond the limits, you can go up to any maximum GPU size since the memory is directly proportional to the performance.
Which is the best GPU for machine learning?
The GeForce RTX 3080 is the first third-party addition in the series and a great one in the tech market. It has 10 GB GDDR6x type memory which is enough to help you in training the models in no time. Further, the card has a challenging build with a stunning design along with excellent cooling and multi-monitor support.